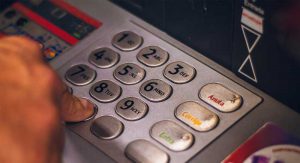
A model to catch them all!
The creation of descriptive and predictive models is based on statistics and recognising patterns in groups with similar characteristics. We have created a methodology that enables detection of anomalies using historical ATM channel transaction behaviour for one of the most important financial institutions in Latin America.
Together with the client and a group of statisticians and technology experts, we created a tool for audit processes that facilitates the detection of anomalies in the fronts that is most important and open to action for the business. From operational issues, availability issues and technology errors to internal or external fraud.
The ATM channel represents an area of business that is subject to direct contact with the public who use it, and is vulnerable for reasons such as connectivity and hardware faults. The number of daily transactions in a network of over 2,000 ATMs involves a huge number of indicators and metrics of technological and operational natures. Currently, a group of auditors is given the task of manually sampling and analysing this data stream to identify risks in the operation of the ATM channel.
The operational characteristics mean that the task of recognising patterns is different for each ATM, as the technology in each unit and the volume of transactions is influenced by factors such as the seasonal phenomenon, demography and even the economic status of the area. To tackle this challenge, /bluetab developed a framework around Python and SQL for segmentation of the most appropriate typologies according to variable criteria, and the detection of anomalies across a set of over 40 key indicators for channel operation. This involved unsupervised learning models and time series that enable us to differentiate between groups of comparable cash machines and achieve more accurate anomaly detection.
The purely mathematical results of this framework were condensed and translated into business-manageable vulnerability metrics, which we developed together with the end user in Qliksense. In this way, we handed the client an analysis environment that covers all the significant operational aspects, but which also enables incorporation of other scenarios on demand.
Now the auditors can analyse months of information considering the temporary market situation, geographic location or technological and transactional characteristics, where they previously only had the capacity to analyse samples.
We are working with our client to drive initiatives to incorporate technology and make the operation more efficient and speed up the response time in case of any incidents.
Patron
Sponsor
© 2024 Bluetab Solutions Group, SL. All rights reserved.